
In today’s world, data science transforms industries with powerful analytics. It reshapes decision-making, driving innovation in healthcare, finance, retail, and transportation. Let’s explore its real-world applications and significant impact.
Understanding the Basics of Data Science
Data science is an interdisciplinary field that combines statistical analysis, machine learning, and computer science to extract meaningful insights and patterns from large datasets. Its importance lies in its ability to uncover hidden relationships, predict future outcomes, and optimize decision-making processes. The results obtained through data science enable organizations to gain a competitive edge, improve efficiency, and achieve their goals effectively.
Defining Data Science and Its Importance
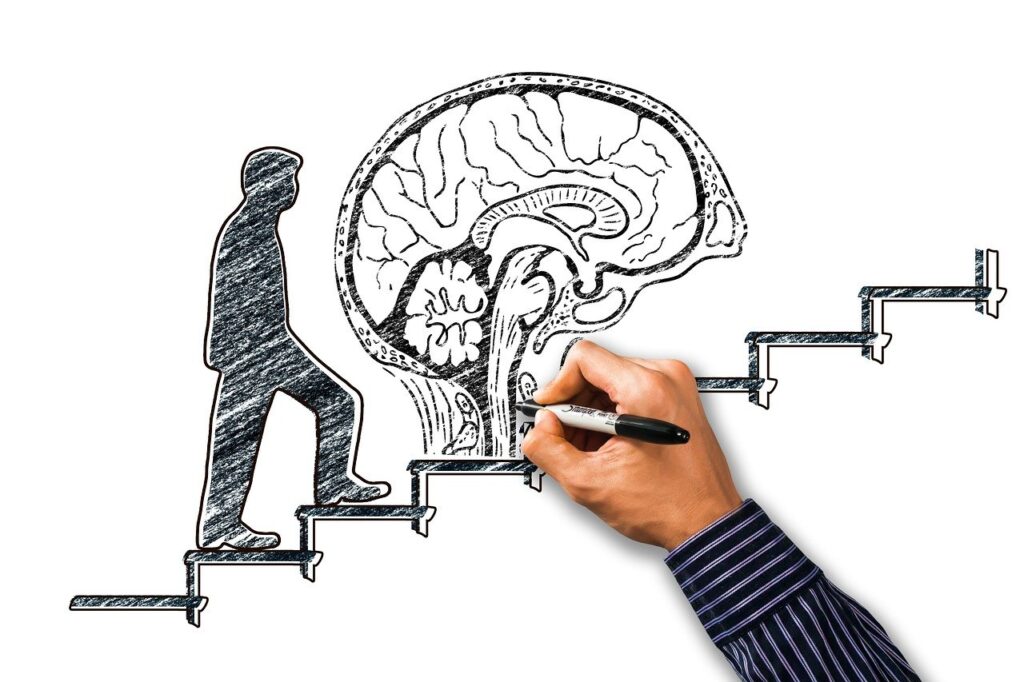
Data science involves a comprehensive approach to analyze and utilize data for innovation. Leveraging advanced techniques, data scientists extract valuable information from overwhelming datasets.
Data science is vital for uncovering hidden patterns and relationships not apparent. Analyzing large datasets helps identify trends, informing decisions and predictions. In healthcare, data science identifies risk factors, predicts outcomes, and improves treatments.
Furthermore, data science plays a crucial role in optimizing decision-making processes. By analyzing historical data and using predictive modeling techniques, organizations can make data-driven decisions that are based on evidence rather than intuition or guesswork. This can lead to more accurate forecasting, better resource allocation, and improved operational efficiency.
Key Components of Data Science
Data science encompasses various key components that work together to derive insights from data. These include:
- Data Collection and Cleansing: Collecting relevant data is the first step in any data science project. Data scientists need to identify and gather the necessary data from various sources, ensuring its quality and accuracy. This involves removing duplicates, handling missing values, and dealing with outliers.
- Exploratory Data Analysis: Once the data is collected, data scientists perform an initial exploration to gain a better understanding of the dataset. This involves summarizing the main characteristics of the data, identifying patterns, trends, and potential relationships, and visualizing the data using charts and graphs.
- Statistical Modeling: Statistical modeling is a fundamental component of data science. It involves applying statistical techniques and models to analyze and interpret the data. This can include hypothesis testing, regression analysis, time series analysis, and more. The goal is to gain insights into the underlying patterns and relationships within the data.
- Machine Learning: Machine learning is a subset of artificial intelligence that focuses on developing algorithms and models that automatically learn and make predictions based on data. This involves training models on historical data and using them to make predictions or classify new data. Machine learning algorithms can be used for tasks such as image recognition, natural language processing, and recommendation systems.
- Data Visualization: Data visualization is the process of creating visual representations that help to understand and communicate the insights derived from data. This can include charts, graphs, maps, and interactive dashboards. Data visualization is important because it allows stakeholders to easily grasp complex information and make informed decisions.
The Role of a Data Scientist
At the heart of data science applications are data scientists, professionals who possess a unique blend of analytical and technical skills. They play a crucial role in leveraging data to solve complex problems, make data-driven decisions, and drive business growth.
Data scientists are proficient in programming languages like Python or R, which are commonly used for data analysis and machine learning. They have expertise in statistical analysis and are familiar with a wide range of statistical techniques and models. Additionally, data scientists possess the ability to communicate and present their findings effectively, as they often need to explain complex concepts to non-technical stakeholders.
In addition to technical skills, data scientists also need to have a strong understanding of the business domain they are working in. This allows them to ask the right questions, identify relevant variables, and translate data insights into actionable recommendations. Data scientists often collaborate with domain experts, business analysts, and other stakeholders to ensure that the insights derived from data align with the organization’s goals and objectives.
In conclusion, data science is a rapidly growing field that has the potential to revolutionize industries and drive innovation. By leveraging advanced analytical techniques and algorithms, data scientists are able to extract valuable insights from data that can be used to make informed decisions, optimize processes, and achieve business objectives.
Data Science in Healthcare
Data science is revolutionizing healthcare by enabling predictive analytics, which can play a vital role in improving patient care. By analyzing large volumes of patient data, including medical records, lab results, and clinical notes, data science algorithms can predict the likelihood of diseases, identify high-risk patients, and recommend personalized treatment plans. This proactive approach helps healthcare providers deliver timely interventions, prevent complications, and ultimately save lives.
For example, imagine a scenario where a patient visits their primary care physician complaining of persistent headaches. Through data science algorithms, the physician can analyze the patient’s medical history, lifestyle factors, and genetic predispositions to predict the likelihood of a serious underlying condition, such as a brain tumor. This early detection allows for prompt referral to a specialist and the initiation of appropriate treatment, potentially saving the patient’s life.
Medical Imaging and Genomics
Medical imaging and genomics generate vast amounts of data that hold immense potential in diagnosis and treatment. Data science algorithms can extract meaningful information from medical images, such as X-rays and MRIs, facilitating early detection of diseases and accurate interpretation of results. Moreover, analyzing genomic data can offer insights into a patient’s genetic predispositions, aiding in personalized medicine and targeted therapies.
Consider the field of radiology, where data science is transforming the way medical images are analyzed. Through advanced image recognition algorithms, data scientists can assist radiologists in identifying subtle abnormalities that may be indicative of diseases such as cancer. This collaboration between humans and machines improves diagnostic accuracy and reduces the chance of misdiagnosis, leading to more effective treatment plans.
Furthermore, the analysis of genomic data has the potential to revolutionize personalized medicine. By studying an individual’s genetic makeup, data scientists can identify specific genetic variants that may influence their response to certain medications. This information can guide healthcare providers in tailoring treatment plans to maximize efficacy and minimize adverse effects, resulting in better patient outcomes.
Drug Discovery and Development
Data science is accelerating the process of drug discovery and development, which traditionally has been time-consuming and expensive. By analyzing complex biological data and conducting virtual screening experiments, data scientists can identify potential drug candidates, predict their efficacy, and optimize the drug development process. This not only reduces costs but also enables the discovery of new treatments for various diseases.
Imagine the arduous process of developing a new drug to combat a deadly infectious disease. Traditionally, the process involved years of lab trial and error. Researchers tested thousands of compounds to find a potential candidate. Now, with data science, scientists use machine learning to analyze databases. They examine chemical structures and biological activity data for efficiency.
This accelerates drug discovery, potentially saving countless lives.
In addition to expediting the drug discovery process, data science also plays a crucial role in optimizing the formulation and dosage of medications. By analyzing patient data, including demographics, genetic information, and medical history, data scientists can identify factors that may affect drug absorption, metabolism, and response. This knowledge enables healthcare providers to prescribe medications at the right dosage and formulation, ensuring maximum efficacy and minimizing the risk of adverse reactions.
In conclusion, data science is revolutionizing healthcare in various ways. From predictive analytics for patient care to medical imaging and genomics, and drug discovery and development, data science is transforming the healthcare landscape. By harnessing the power of data and advanced algorithms, healthcare providers can deliver more personalized, efficient, and effective care to patients, ultimately improving outcomes and saving lives.
Data Science in Finance
Data science is revolutionizing the financial industry, bringing about significant advancements in various areas. From fraud detection and prevention to risk management and investment strategies, data science is playing a crucial role in shaping the future of finance.
Fraud Detection and Prevention
One of the key applications of data science in finance is in fraud detection and prevention. With the increasing complexity of fraudulent activities, traditional rule-based systems are no longer sufficient. Data scientists leverage advanced analytics techniques to analyze transactional data and customer behavior patterns, enabling the development of intelligent fraud detection systems. These systems can flag suspicious activities in real-time, helping financial institutions safeguard their assets, protect customer interests, and maintain the integrity of the financial ecosystem.
By continuously monitoring and analyzing large volumes of data, data scientists can identify patterns and anomalies that indicate potential fraudulent activities. They can also develop machine learning models that learn from past incidents and adapt to new fraud patterns, making the detection process more accurate and efficient.
Risk Management and Investment Strategies
Data science is also revolutionizing risk management and investment strategies in the financial industry. By analyzing market trends, historical data, and economic indicators, data scientists can provide valuable insights that help financial institutions make informed decisions.
Using predictive models and machine learning, data scientists forecast risks and opportunities. Financial institutions optimize portfolio allocations based on these predictions. Understanding underlying data patterns helps identify high-return, low-risk investment opportunities. This not only enhances returns on investments but also minimizes financial losses.
Customer Segmentation and Personalized Marketing
Data science is transforming customer segmentation and enabling personalized marketing campaigns in the finance industry. By analyzing vast amounts of customer data, including demographics, browsing behavior, and purchase history, data scientists can segment customers into distinct groups based on their preferences and characteristics.
This segmentation allows financial institutions to tailor their marketing strategies and deliver personalized product recommendations to each customer segment. By understanding the needs and preferences of different customer groups, businesses can create targeted advertisements and marketing campaigns that resonate with their audience. This results in better customer engagement, increased conversion rates, and ultimately, higher customer satisfaction.
Furthermore, data science enables financial institutions to leverage customer data to identify cross-selling and upselling opportunities. By analyzing the purchasing patterns of customers, data scientists can identify products or services that are likely to be of interest to specific customer segments. This allows businesses to offer personalized recommendations and promotions, increasing the likelihood of additional sales and revenue.
In conclusion, data science is revolutionizing the finance industry by providing valuable insights and enabling advanced analytics techniques. From fraud detection and risk management to personalized marketing, data science is reshaping the way financial institutions operate. As technology continues to advance, the role of data science in finance is only expected to grow, bringing about further advancements and opportunities.
Data Science in Retail
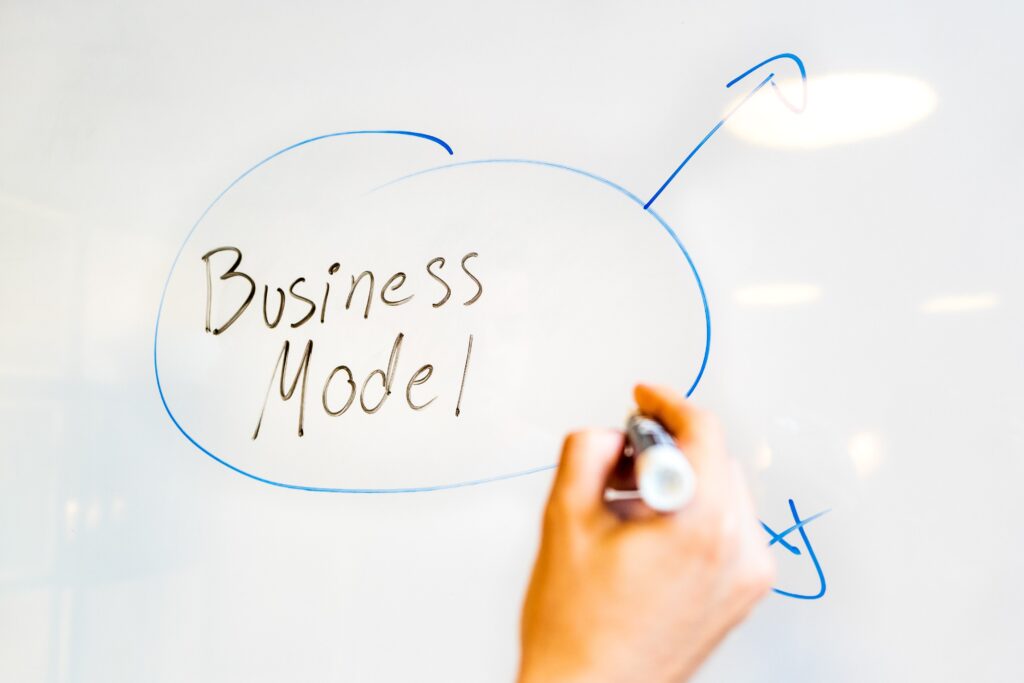
Data science is revolutionizing the retail industry by providing valuable insights and solutions to various aspects of the business. From inventory management to customer behavior analysis, data science is transforming the way retailers operate and interact with their customers.
Inventory Management and Demand Forecasting
One of the key areas where data science is making a significant impact in retail is inventory management and demand forecasting. By leveraging advanced analytics techniques, data scientists can analyze historical sales data, market trends, and external factors to accurately predict future demand patterns. This enables retailers to adjust their inventory levels accordingly, minimizing the risk of overstocking or understocking. By optimizing inventory management, retailers can reduce costs associated with excess inventory while ensuring that popular products are always available to customers. This not only improves customer satisfaction but also maximizes profitability for the business.
Customer Behavior Analysis
Understanding customer behavior and preferences is crucial for retailers to make informed decisions and provide personalized experiences. Data science plays a vital role in analyzing customer data, such as purchase history, browsing habits, and social media interactions. By applying advanced analytics algorithms, data scientists can uncover valuable insights into customer motivations, preferences, and shopping habits. Armed with information, retailers tailor product offerings, marketing, and pricing. By identifying behavior patterns, they send targeted promotions, enhancing the shopping experience and fostering customer loyalty.
Enhancing the Shopping Experience
Data science is also instrumental in enhancing the overall shopping experience for customers. Leveraging customer data, retailers enhance shopping journeys with recommendation systems and personalized ads. These systems analyze preferences and past purchases, offering targeted recommendations. This personalized approach simplifies the discovery of new, potentially interesting items.
Personalized advertisements, based on customer demographics and browsing history, can deliver tailored offers and discounts, further enhancing the shopping experience. By leveraging data science, retailers can create a unique and satisfying shopping journey that keeps customers engaged and coming back for more.
In conclusion, data science transforms retail by offering insights and solutions. It optimizes inventory, forecasts demand, and analyzes customer behavior. This empowers retailers to make data-driven decisions and stay competitive. As technology advances, data science’s role in retail becomes even more critical. It enables retailers to thrive and deliver exceptional customer experiences.
Data Science in Transportation
Optimizing Routes and Schedules
Data science is revolutionizing the transportation industry by optimizing routes and schedules. By analyzing historical data, traffic patterns, and external factors such as weather and events, data scientists can develop algorithms that suggest the most efficient routes, minimize travel time, and reduce fuel consumption. This not only improves efficiency but also enhances customer satisfaction and reduces carbon emissions.
Predictive Maintenance for Vehicles
Data science enables predictive maintenance for vehicles, helping to prevent breakdowns and minimize downtime. By analyzing sensor data and historical maintenance records, data scientists predict maintenance needs. Proactive interventions reduce the risk of unexpected failures, ensuring vehicle safety. It also optimizes maintenance schedules, resulting in cost savings for transportation companies.
Enhancing Safety and Efficiency
Utilizing data science, transportation companies can enhance safety and efficiency in multiple ways. By analyzing traffic data, road conditions, and driver behavior, data scientists can identify areas prone to accidents and develop strategies to reduce risks. Data-driven optimization of fleet management, resource allocation, and route planning further improves operational efficiency, reduces costs, and enhances safety for both passengers and drivers.
Future Trends in Data Science
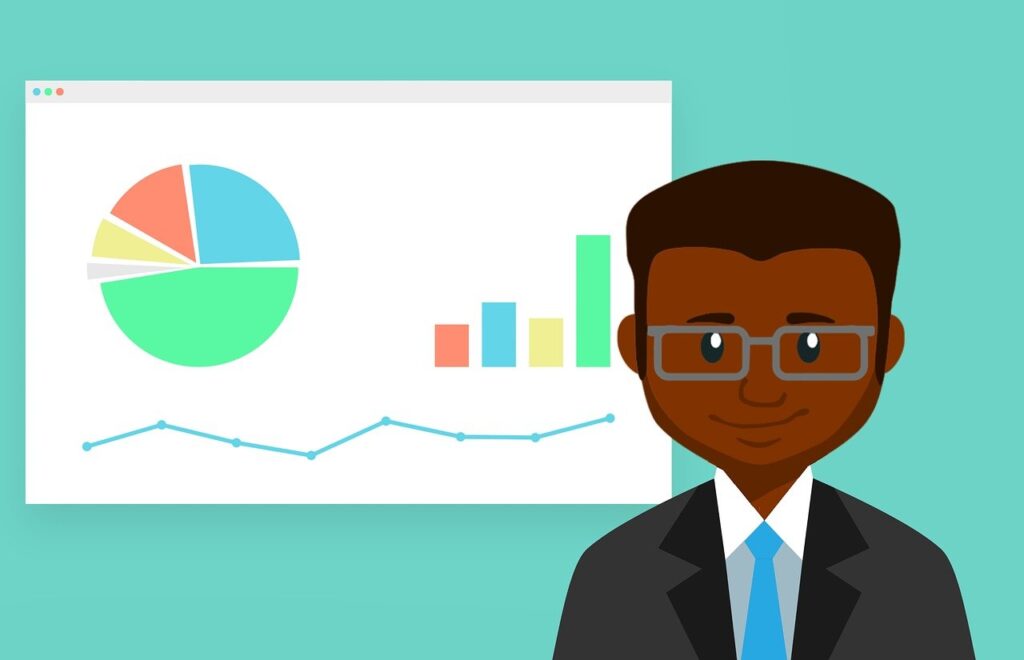
The Rise of Automated Data Science
As data science continues to evolve, automation is becoming a significant trend. The rise of automated data science platforms and tools allows organizations to leverage the power of data science without requiring extensive expertise. These platforms automate data cleaning, model selection, and hyperparameter tuning, making data science more accessible and efficient.
Data Science and Artificial Intelligence
Data science and artificial intelligence (AI) go hand in hand. AI techniques, such as machine learning and natural language processing, are powered by the insights derived from data science. With the increasing availability of data and advancements in AI algorithms, data science will continue to drive the development and application of AI technologies across industries.

Ethical Considerations in Data Science
As data science becomes widespread, ethical considerations are crucial. Organizations must ensure data’s ethical use, maintain privacy, avoid biases, and prevent discrimination in algorithmic decision-making. Data scientists play a vital role in promoting ethics, developing transparent, accountable models to mitigate risks, and maximize societal benefits.
In conclusion, data science applications revolutionize diverse industries by leveraging data. From healthcare and finance to retail and transportation, data science’s impact is undeniable. As technology advances and industries embrace data-driven decisions, the potential for data science to transform businesses and improve lives is limitless.