In today’s data-driven world, data analytics is essential for businesses to gain insights and make informed decisions. With the rapid advancements in technology, the field of data analytics continues to evolve, offering new opportunities and challenges. This article will delve into the latest trends in data analytics. It will explore its definition, importance, evolution, current trends, future possibilities, challenges, and opportunities.
Understanding Data Analytics
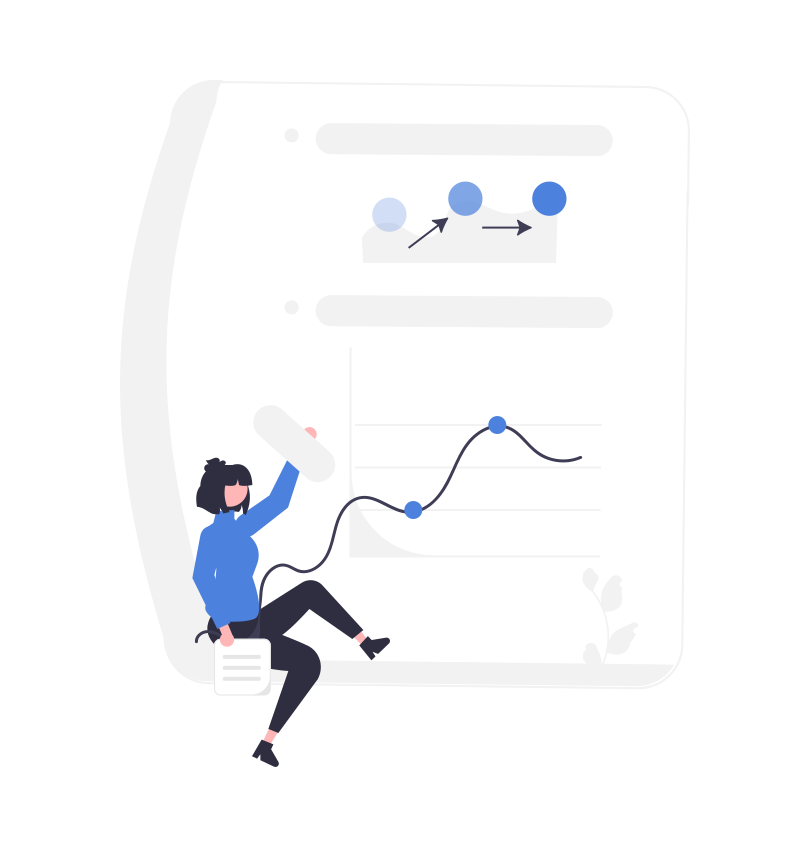
Definition and Importance of Data Analytics
Data analytics involves examining, cleaning, transforming, and modeling data. This aids in discovering information and supporting decision-making. It involves using various techniques and tools to analyze structured and unstructured data, extract patterns, and identify trends.
Data analytics is crucial for businesses as it enables them to gain valuable insights and make data-driven decisions. By analyzing data, organizations can understand customer behavior, identify market trends, optimize processes, improve operational efficiency, and drive innovation. In today’s competitive landscape, data analytics has become a strategic imperative for businesses looking to stay ahead.
Let’s delve deeper into the world of data analytics. We’ll explore key techniques and tools in this field.
The Role of Data Analytics in Business Decision Making
Data analytics plays a vital role in business decision-making processes. By analyzing historical and real-time data, organizations can make informed decisions that are backed by data-driven insights. Data analytics helps organizations identify areas for improvement, discover new opportunities, and mitigate risks.
One of the techniques commonly used in data analytics is descriptive analytics. This involves analyzing historical data to understand what has happened in the past. By examining trends and patterns, organizations can gain a better understanding of their business operations and customer behavior.
Another important technique is diagnostic analytics, which focuses on understanding why certain events occurred. By drilling down into the data, organizations can identify the root causes of problems or successes. This enables corrective actions.
Predictive and Prescriptive Analytics Models
Data analytics enables businesses to implement predictive and prescriptive analytics models. These provide forward-looking insights and recommendations.Predictive analytics uses historical data and statistical algorithms to forecast future trends and outcomes. This empowers organizations to anticipate future trends, forecast demand, optimize pricing, and make proactive decisions that drive business growth.
Prescriptive analytics takes predictive analytics a step further. It offers recommendations for achieving desired outcomes. By considering various scenarios and constraints, organizations can make informed decisions that maximize their chances of success.
In addition to these techniques, data analytics relies on a variety of tools and technologies. These include data visualization tools, which help present complex data in a visually appealing and easy-to-understand format. By using charts, graphs, and dashboards, organizations can communicate insights effectively and facilitate decision-making.

Furthermore, machine learning and artificial intelligence algorithms are increasingly used in data analytics. They automate processes and uncover hidden patterns. These algorithms can analyze vast data with speed and accuracy surpassing human capabilities. This enables deeper insights and predictions.
In conclusion, data analytics is a powerful tool. It enables businesses to extract valuable insights from data and make informed decisions. By leveraging various techniques and tools, organizations can gain a competitive edge in today’s data-driven world. Whether it’s understanding customer behavior or identifying market trends, data analytics plays a crucial role. It’s indispensable for decision-making.
The Evolution of Data Analytics
Data analytics has come a long way from traditional methods. In the past, organizations relied on basic analytics to analyze structured data in spreadsheet formats. However, with the advent of big data, organizations deal with vast data volumes. This data comes from various sources.
Big data analytics involves using advanced technologies and techniques to analyze large and complex datasets. It includes technologies like Hadoop and Apache Spark. These enable organizations to process, store, and analyze massive data efficiently. Big data analytics allows businesses to uncover hidden patterns, correlations, and insights that were previously difficult to identify.
With the rise of big data, new roles have emerged in data analytics. These roles include data scientists, data engineers, and data analysts. These professionals are in high demand as organizations seek expertise in managing and analyzing large datasets.
Moreover, the field of data analytics has expanded beyond just structured data. Unstructured data, including text documents, images, and videos, can now be analyzed. This is achieved using NLP and computer vision techniques. This has opened up new possibilities for organizations to gain insights from diverse data sources.
The Impact of AI and Machine Learning on Data Analytics
The integration of artificial intelligence (AI) and machine learning (ML) has revolutionized the field of data analytics. AI and ML algorithms can analyze large datasets and learn from patterns to make accurate predictions and recommendations.
Machine learning algorithms can identify patterns in data and make predictions based on historical records. This enables organizations to automate decision-making processes, optimize resource allocation, detect anomalies, and personalize customer experiences. AI-powered analytics tools can also automate data cleansing, data integration, and data visualization tasks, saving time and improving efficiency.
Furthermore, AI and ML have enabled the development of predictive analytics models that can forecast future trends and outcomes. These models can help organizations make informed decisions and take proactive measures to stay ahead in the competitive market.
AI and ML in Fraud Detection
Another area where AI and ML have made a significant impact is in the field of fraud detection. By analyzing large transactional data volumes and identifying fraudulent behavior patterns, AI-powered analytics systems help organizations detect and prevent fraud.
In addition to AI and ML, data analytics is enhanced by emerging technologies like natural language processing (NLP). NLP enables computers to understand and interpret human language. This technology is particularly useful in analyzing customer feedback, social media posts, and other forms of unstructured data.
Overall, the evolution of data analytics is driven by technology, big data availability, and AI and ML integration. Organizations collect vast data, propelling analytics’ growth and facilitating data-driven decisions.
Current Trends in Data Analytics
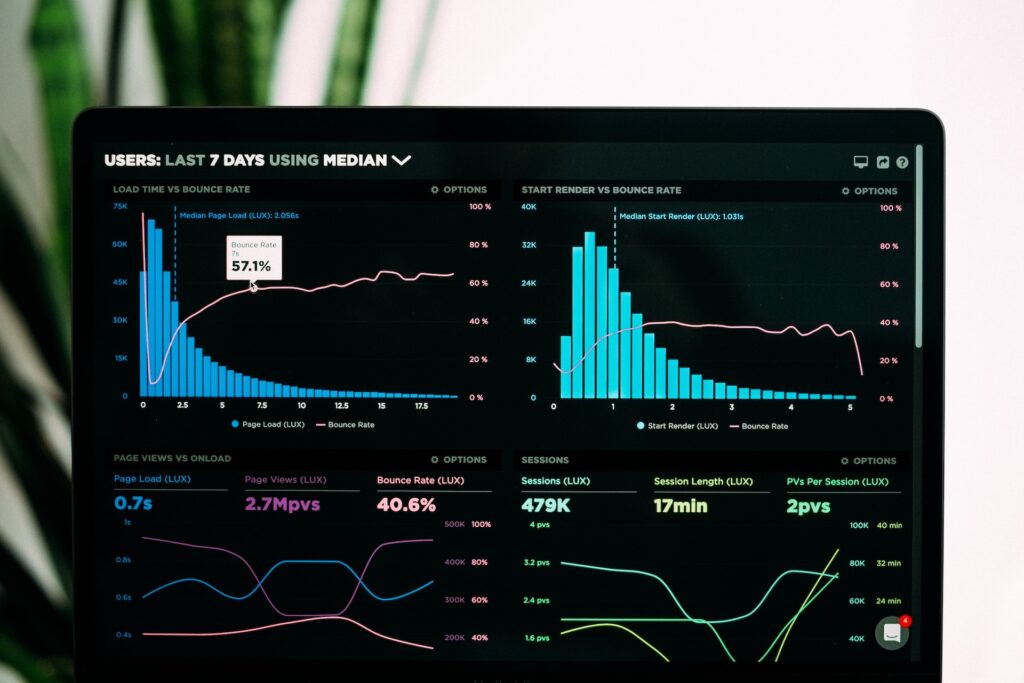
Data analytics is a rapidly evolving field, with new trends and technologies emerging all the time. In this article, we will explore some of the current trends in data analytics that are shaping the industry and revolutionizing the way organizations make decisions.
Predictive and Prescriptive Analytics
Predictive analytics involves using historical and real-time data to forecast future outcomes and events. By applying statistical algorithms and machine learning techniques, organizations can make accurate predictions about customer behavior, demand patterns, and market trends. Predictive analytics empowers businesses to take proactive measures to capitalize on opportunities and mitigate risks.
Prescriptive analytics takes predictive analytics a step further by providing actionable insights and recommendations. It goes beyond predicting what is likely to happen and provides organizations with guidance on what actions to take to achieve desired outcomes. Prescriptive analytics helps businesses optimize decisions, prioritize resources, and enhance operational effectiveness.
For example, a retail company can use predictive analytics to forecast customer demand for certain products during different seasons. Based on these predictions, they can then use prescriptive analytics to determine the optimal inventory levels and pricing strategies to maximize sales and minimize stockouts.
Real-time Analytics
Real-time analytics refers to analyzing data as it is generated to provide insights and make decisions in real-time. With the increasing volume and velocity of data, organizations need to analyze data instantaneously to gain a competitive edge. Real-time analytics allows businesses to monitor operational performance, detect anomalies, and respond quickly to changing conditions.
Real-time analytics is particularly valuable in industries such as finance, e-commerce, healthcare, and supply chain management where immediate decision-making is critical. For example, a financial institution can use real-time analytics to detect fraudulent transactions and take immediate action to prevent financial losses.
By leveraging real-time data analytics, organizations can optimize processes, improve customer experiences, and gain a competitive advantage. For instance, an e-commerce company can use real-time analytics to personalize product recommendations for each customer based on their browsing behavior and purchase history.
Data Automation
Data automation is another trend that is transforming the field of data analytics. Automation involves using technologies and algorithms to streamline and expedite data-related processes. From data collection and cleansing to analysis and reporting, automation enables organizations to reduce manual effort and improve accuracy.
Automation also allows businesses to scale their analytics capabilities by handling large volumes of data efficiently. By automating repetitive tasks, organizations can free up resources to focus on more value-added activities such as data interpretation, strategy formulation, and decision-making.
For example, a healthcare provider can automate the process of extracting patient data from electronic health records and analyzing it to identify patterns and trends. This automation not only saves time and effort but also improves the accuracy and reliability of the analysis.
In conclusion, predictive and prescriptive analytics, real-time analytics, and data automation are some of the key trends in data analytics that are driving innovation and transforming the way organizations leverage data. By embracing these trends, businesses can gain valuable insights, make informed decisions, and stay ahead in today’s data-driven world.
The Future of Data Analytics
Data analytics has become an integral part of decision-making in various industries. As technology continues to advance, new trends and innovations are shaping the future of data analytics. In this article, we will explore two emerging trends that are set to transform the field of data analytics.
The Rise of Augmented Analytics
Augmented analytics is an exciting trend that combines data analytics with natural language processing (NLP) and machine learning capabilities. This powerful combination aims to democratize data analytics by providing business users with self-service analytics tools that are easy to use and understand.
With augmented analytics tools, business users can now explore data, create visualizations, and generate insights without relying heavily on data scientists or analysts. This empowers organizations to foster a data-driven culture, where decision-making is based on accurate and timely insights.
One of the key advantages of augmented analytics is its ability to automate data preparation, analysis, and visualization tasks. By automating these processes, augmented analytics accelerates the decision-making process and enables organizations to make informed choices quickly.
Furthermore, augmented analytics tools leverage natural language processing to enable users to interact with data in a more intuitive and conversational manner. This eliminates the need for complex coding or technical skills, making data analytics accessible to a wider audience.
The Potential of Quantum Computing in Data Analytics
Quantum computing is another groundbreaking technology that has the potential to revolutionize data analytics. Unlike classical computers, which process information using bits, quantum computers use quantum bits or qubits to perform computations.
Quantum computing offers immense computational power and can process vast amounts of data simultaneously. This capability makes it particularly well-suited for solving complex optimization and simulation problems that are often encountered in data analytics.
Industries such as finance, healthcare, and logistics, which deal with data-intensive operations, can greatly benefit from the potential of quantum computing. For example, in finance, quantum computing can be used to optimize investment portfolios, detect fraud, and predict market trends with greater accuracy.
In healthcare, quantum computing can aid in drug discovery by simulating molecular interactions and identifying potential drug candidates more efficiently. Additionally, in logistics, quantum computing can optimize supply chain networks, reducing costs and improving efficiency.
Furthermore, quantum computing has the potential to uncover novel patterns and correlations in data that may have been previously overlooked. This can lead to groundbreaking discoveries and insights that can drive innovation in various industries.
While quantum computing is still in its early stages, researchers and organizations are actively exploring its potential applications in data analytics. As the technology continues to advance, we can expect to see quantum computing playing a significant role in shaping the future of data analytics.
Challenges and Opportunities in Data Analytics
Data Privacy and Security Concerns
While data analytics presents several opportunities, it also brings challenges, particularly concerning data privacy and security. With the increasing volume and variety of data being collected, organizations need to ensure that data is handled securely and in compliance with privacy regulations.
Data breaches and cyber threats pose significant risks to organizations and can lead to reputational damage, legal repercussions, and financial losses. To address these challenges, organizations need to invest in robust data security measures, employee training, and data governance frameworks to protect sensitive information and build trust with customers.
One example of a data breach that had severe consequences for an organization is the Equifax data breach in 2017. The breach exposed the personal information of approximately 147 million people, including their names, Social Security numbers, birth dates, addresses, and in some cases, driver’s license numbers. This breach not only resulted in financial losses for Equifax but also damaged their reputation and eroded customer trust.
To prevent such incidents, organizations must implement strong encryption protocols, multi-factor authentication, and regular security audits. Additionally, they should educate employees about the importance of data security and train them on best practices for handling sensitive information.
The Skills Gap in Data Analytics
Another challenge in data analytics is the shortage of skilled professionals. As the demand for data analytics grows, there is a shortage of data scientists, data engineers, and other analytics professionals who possess the necessary technical and analytical skills.
To bridge the skills gap, organizations need to invest in training and upskilling programs to develop a workforce that is proficient in data analytics. Collaboration between academia and industry is also essential to nurture the next generation of data analytics talent.
One initiative aimed at addressing the skills gap is the establishment of data science boot camps and online courses. These programs provide intensive training in data analytics and help individuals acquire the necessary skills to enter the field. Additionally, universities are expanding their data science programs and offering specialized degrees to meet the growing demand for skilled professionals.
Furthermore, organizations can implement mentorship programs to pair experienced data analytics professionals with aspiring individuals. This mentorship can provide valuable guidance and support, helping newcomers navigate the complexities of the field and accelerate their learning.
Opportunities for Businesses in Data Analytics
Amidst the challenges, data analytics presents significant opportunities for businesses. By harnessing the power of data, organizations can gain a competitive advantage, drive innovation, and improve operational efficiency.
Businesses can leverage data analytics to personalize customer experiences, optimize marketing campaigns, improve supply chain management, implement predictive maintenance, and enhance decision-making processes. By embracing data analytics, organizations can uncover valuable business insights and unlock new opportunities for growth.
For example, a retail company can use data analytics to analyze customer purchasing patterns and preferences, allowing them to tailor personalized recommendations and offers. This level of personalization can significantly enhance customer satisfaction and loyalty, leading to increased sales and revenue.
Furthermore, data analytics can help organizations identify inefficiencies in their operations and make data-driven decisions to improve productivity. By analyzing data on production processes, supply chain logistics, and resource allocation, businesses can identify bottlenecks and implement targeted improvements to streamline operations and reduce costs.
In conclusion, as data continues to proliferate, the field of data analytics is constantly evolving. The latest trends in data analytics, such as predictive and prescriptive analytics, real-time analytics, and data automation, offer businesses immense opportunities to gain insights, drive innovation, and improve decision-making processes. However, challenges such as data privacy and security concerns and the skills gap need to be addressed for organizations to fully leverage the power of data analytics. Looking ahead, the rise of augmented analytics and the potential of quantum computing hold exciting possibilities for the future of data analytics. By staying up-to-date with the latest trends and embracing data analytics, businesses can position themselves for success in this data-driven era.